Picky-shy model of dating apps
This is the final post (at least for the foreseeable future) on the statistical physics of dating apps [1]. In this post we will introduce two types of the users: some of them will be picky (giving likes predominantly to more attractive users), while some of them will be shy (giving likes predominantly to less attractive users). Who is more successful?
Replacement model of dating apps
In this post I continue my exploration of the [1]. This time let us consider what happens if the users who were matched are replaced by a random sample from the population. Will only the least and most attractive ones remain unmatched?
Was 2022 as good as 2021?
Well, at least it was as productive on Physics of Risk blog. Though with less focus with quite a few assorted posts. In a few a bit longer series of posts we have taken a look at three papers I have found interesting. [1] inspired few posts on traffic. [2] inspired couple posts on biology. Exploration of [3] continues in series of posts on dating apps.
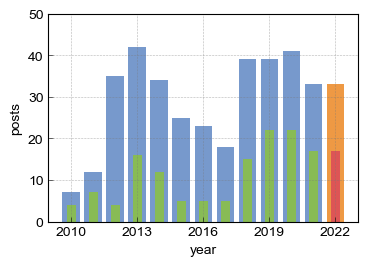
Once again, at the time of writing this post, I still do not have an idea what we will have in store for 2023. Obviously, we will finish dating apps series. And then we will have a few more assorted posts?
References
- P. L. Krapivsky, S. Redner. Where should you park your car? The 1/2 rule. Journal of Statistical Mechanics 2020: 073404 (2020). arXiv:2003.10603 [physics.soc-ph]. doi: 10.1088/1742-5468/ab96b7.
- P. L. Krapivsky, S. Redner. Birds on a Wire. arXiv:2205.00995 [cond-mat.stat-mech].
- F. Olmeda. Towards a statistical physics of dating apps. arXiv:2107.14076 [physics.soc-ph].
Dropout model of dating apps
In this post I continue my exploration of the [1]. In this post I will consider what happens if the users decide to dropout from the app as soon as they are matched with somebody.